Generative AI
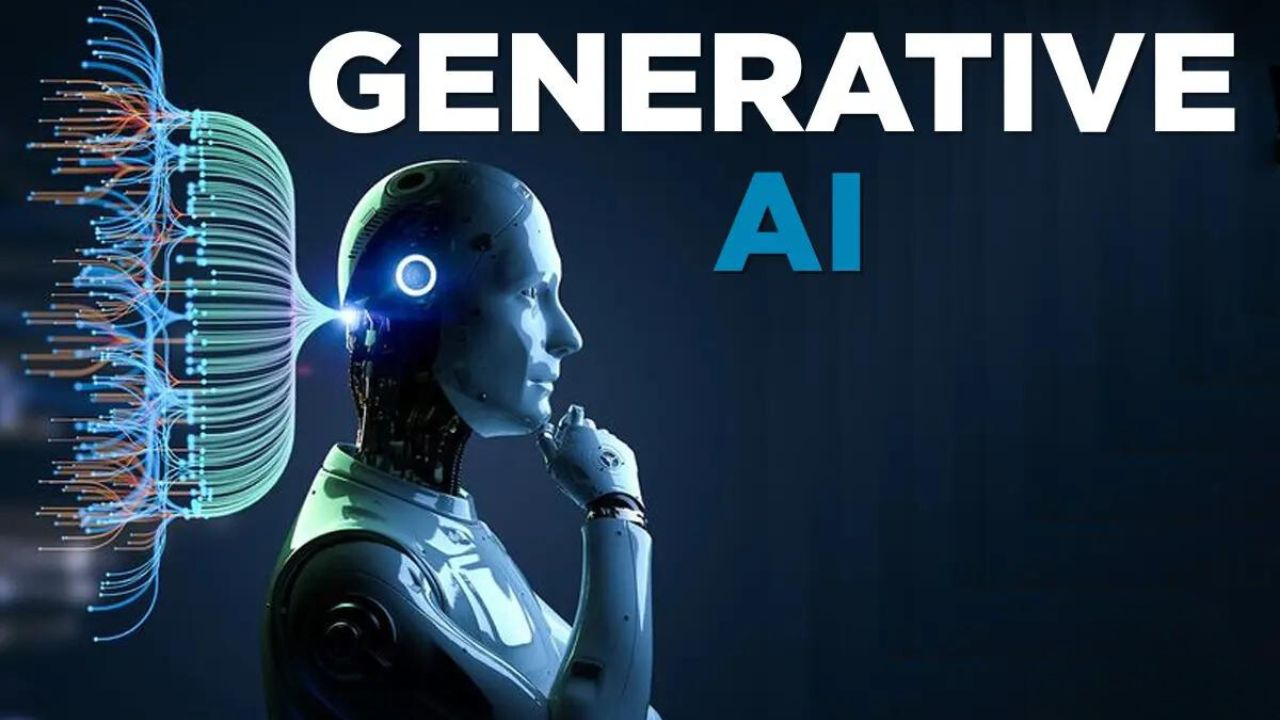
Generative AI refers to a class of artificial intelligence systems designed to generate new, original content. These systems use various techniques, including machine learning and deep learning, to produce data that is similar to but not an exact copy of the input data they were trained on. One notable example of generative AI is the previously mentioned Generative Adversarial Networks (GANs).
Key points about generative AI:
- Variety of Outputs: Generative AI can be applied to different types of data, such as images, text, music, and more. It’s not limited to a specific domain, allowing for diverse applications.
- Learning Patterns: These systems learn patterns and structures from large datasets during training. Once trained, they can generate new instances that share characteristics with the training data.
- Conditional Generation: Some generative AI models can be conditioned on specific inputs or constraints, allowing users to influence the output based on desired characteristics.
- Creative Applications: Generative AI is used in creative fields, such as art, music, and design, to automatically generate new and unique content. It has also found applications in areas like drug discovery and content creation for video games.
- Challenges: Ethical considerations and potential misuse are challenges associated with generative AI, especially when it comes to creating deepfakes or generating content that could be harmful or misleading.
- Examples: Besides GANs, other generative AI models include Variational Autoencoders (VAEs), autoregressive models, and reinforcement learning-based generators.
Generative AI has shown significant advancements and has the potential to impact various industries, but careful consideration of ethical implications and responsible use is crucial as the technology continues to evolve.